Applying Learning Analytics to Inform Online Practices: Interaction and Presence in Case-Based Discussions
Concurrent Session 5
Brief Abstract
This study used learning analytics to inform high and low levels of interactions. The findings will help design thinkers, faculty, training professionals, and researchers predict students’ learning in case-based discussions and help increase sense of presence. The results of this study are beneficial for any institutions and any audience level.
Presenters
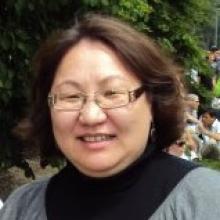
Extended Abstract
Context: Case-based discussions has become a popular approach to facilitate meaningful learning through application of real-world scenarios (Ertmer & Koehler, 2014). Through meaningful interactions, students engage in solving problems through analysis of the issues, consideration of underlying principles, development of solutions, and reflection on the problem solving process (Smith & Ragan, 2005). Student interaction is the key to success in case-based discussions because it involves a two-way communication. By interacting with each other, students can get feedback, exchange and construct ideas, and increases achievement (Anderson, 2003). Research on online learning report that interaction is a strong predictor of student satisfaction and effective learning (Kuo et al., 2013). Huss, Sela, and Eastep (2015) found communication through discussions is essential to students’ success in online courses. Richardson and Ice (2010) found that discussions initiated with specific case resulted in sustained learner interactions that engender critical thinking. This study used learning analytics to inform how case-based discussions influenced student interactions, levels of cognitive presence, and how students perceived presence in case-based discussions.
Questions: 1) Were there any differences in interactions between students with high and low levels of interaction in case-based discussions? 2) How was cognitive presence expressed by students with high and low levels of interaction in case-based discussions? and 3) Were there any differences between students with high and low levels of interaction and their perceptions of online presence (teaching, social and cognitive) in case-based discussions?
Methods: A mixed methods case study was used to provide an in-depth description and analysis regarding how cognitive presence was expressed in case-based discussions. This study explored whether students were different in their interaction and perceived presence in case-based discussions. Twenty-four graduate students (9 males and 15 females) enrolled in an online master’s program in Curriculum and Educational Technology participated in this study. The students ranged in age from twenty-one to forty-five years. Most (n=19) of them had taken three or more online courses prior to participating in this study. Students participated in required eight discussions on various topics on instructional design. For this research, two case-based discussions in weeks 3 and 4 were selected.
Qualitative and quantitative data were collected via Blackboard. Quantitative data from discussion posts (n=496) were collected including the number of responses to peers (n=312) and the number of responses received from peers (n=184). We quantified responses by using degree centrality as an indicator of student interaction. Kim, Park, Yoon, & Jo (2016) validated the accuracy of degree centrality as a proxy variable of the prediction model. Students were divided by high (n=14) and low (n=10) levels of interactions. In-degree centrality (IDC) when students received responses and out-degree centrality (ODC) when students sent responses were analyzed by counting the connections each had established with peers. IDC was calculated as total number of replies received by students and divided by the number of students minus one (n-1). ODC was calculated as total number of replies students sent to others divided by the number of students minus one (n-1). Students in the range of average over 0.20 were considered as high-level interaction and students with average lower than 0.19 were identified as low-level of interaction.
Qualitative data were collected from discussion postings and coded by indicators identified by the Practical Inquiry Model. The procedure involved segmenting postings into meaningful units, categorizing units into one of the four phases of cognitive presence, and summing the frequency of units in each phase. In total, 346 segments including 150 segments in week 3 and 196 segments in week 4 were analyzed. Non-substantive appreciation postings (n=150) were not coded. Inter-ratter reliability was established using a consensus approach for coding the transcripts.
Additional quantitative data were obtained through students’ responses (n=21) to the Community of Inquiry survey about teaching, social and cognitive presence. The survey included 34 items on a 5-point Likert Scale (from Strongly Disagree to Strongly Agree). The survey was validated with Cronbach alpha 0.91 for social presence, 0.95 for cognitive presence, and 0.94 for teaching presence (Arbaugh et al., 2008).
Descriptive statistics was applied to analyze data in this study for all research questions because of a small sample size (n=24).
Results and Discussion:
RQ1: The results revealed that in both discussions all students sent out more responses to their peers (ODC 0.28) than they received responses from others (IDC 0.17). When differences between high and low levels of interaction were examined, the results were consistent across both discussions. Students at high level of interaction sent out (ODC 0.34) and received (IDC 0.22) more responses to compare with students at low level of interaction (ODC 0.21 and IDC 0.10). The results were also consistent for both discussions across two levels of interactions. It is not surprising that active students participated more in both discussions. We also checked students’ demographic on gender, age, and the number online courses previously taken to understand what type of students were active. We did not find any consistency or differences based on students’ demographic data to explain students’ online communicative behavior.
RQ2: The results revealed that overall posts at exploration (41.62%) and integration (31.79%) levels of cognitive presence expressed more frequently to compare with posts at triggering events (12.43%) or resolution (5.20%). To find differences at the levels of cognitive presence between students at high and low levels of interaction, we combined triggering events and exploration levels together and labelled them as low level of cognitive presence. We also combined integration and resolution levels together and labelled them as high level of cognitive presence.
The findings for the first discussion showed that there were no differences in percentages at high level of cognitive presence across both interaction levels. This means that all students participated and posted at high level of cognitive presence. However, students at high level of interaction had higher percentages at low level of cognitive presence than students at low level of interaction in the first discussion. This means that students at high level of interaction explored more information and shared it with peers or they asked more questions. The findings for the second discussion revealed that students at high level of interaction had higher percentages at both levels of cognitive presence than students at low level of interaction. However, the posts at low level of cognitive presence had a slight difference between both levels of interaction.
RQ3: The survey results revealed that students had a higher perception of teaching presence (M=4.38, SD=0.20). Overall, students rated design and organization (M=4.62, SD =0.49) higher than facilitation (M=4.17, SD=0.79) or direct instruction (M=4.48, SD=0.54). Cognitive presence sections exploration (M=4.24, SD=0.50), integration (M=4.24, SD=0.54) and resolution (M=4.24, SD=0.51) surprisingly received the same ratings while triggering event rated lower (M=4.13, SD=0.41). Social presence received the lowest score (M=3.99, SD=0.11). This means that even case-based discussions impacted students’ perceived learning, it did not help to develop the sense of community.
The results on differences between high and low levels of interactions revealed that students at low level of interaction rated teaching presence higher (M=4.45, SD=0.61) than students at high level (M=4.41, SD=0.62). There were differences in students’ rating of teaching presence items. The item on whether instructor actions reinforced the development of a sense of community among discussion participants received the lowest rating among students at low level of interaction (M=4.00, SD=1.07). The item on whether instructor was helpful in identifying areas of agreement and disagreement during discussions rated as the lowest among students at high level of interaction (M=3.69, SD=1.11).
Cognitive presence received similar rating by both levels of interactions (M=4.21, SD=0.50) showing that case-based discussions was an effective approach to facilitate learning. The item on whether students felt motivated to explore content related questions rated as the lowest among students at high level of interaction. Students at low level of interaction rated the items on whether combining new information helped them answer questions (M=4.00, SD=0.93) and whether they can apply the knowledge created in discussions to their work (M=4.00, SD=0.76) as the lowest. These findings showed students at low level of interaction perceived difficulties in participating in discussions. On the contrary, students at high level of interaction did not find the value to explore content related question.
Conclusion: This study found evidence case-based discussions can improve student interactions and engage them into meaningful discourse. However, even discussions proved to be an effective approach, it still did not facilitate the sense of community. Students at low level of interaction seems getting difficulties in participating in discussions where they were required to analyze and synthesize information. However, teaching presence has a greater impact on perceived sense of presence confirming the importance of facilitation and feedback in online discussions.
Presenters will share results and invite the audience to discuss how the findings can be applied to real online courses. The audience will also share their own practices. They will reflect on how their practices can be improved by using the findings of this study. The audience will learn how learning analytics can help predict student learning and sense of presence.
References
Anderson, T. (2003). Getting the mix right again: An updated and theoretical rationale for interaction. The International Review of Research in Open and Distributed Learning, 4(2).
Arbaugh, J.B., Cleveland-Innes, M., Diaz, S.R., Garrison, D.R., Ice, P., Richardson, J.C., & Swan, K.P. (2008). Developing a community of inquiry instrutment: Testing a measure of the Community of Inquiry framework using a multi-institutional sample. Internet and Higher Education, 11(3), 133-136.
Borokhovski, E., Tamim, R., Bernard, R. M., Abrami, P. C., & Sokolovskaya, A. (2012). Are contextual and designed student–student interaction treatments equally effective in distance education? Distance Education, 33(3), 311–329.
Ertmer, P.A., & Koehler, A.A. (2014). Online case-based discussions: Examining coverage of the afforded problem space. Educational Technology Research and Development, 62(5), 617-636.
Huss, J. A., Sela, O., & Eastep, S. (2015). A case study of online instructors and their quest for greater interactivity in their courses: Overcoming the distance in distance education. Australian Journal of Teacher Education, 40(4).
Kim, D., Park, Y., Yoon, M., & Jo, I. H. (2016). Toward evidence-based learning analytics: Using proxy variables to improve asynchronous online discussion environments. The Internet and Higher Education, 30, 30-43.
Kuo, Y. C., Walker, A., Belland, B. R., & Schroder, K. E. E. (2013). A predictive study of student satisfaction in online education programs. International Review of Research in Open and Distance Learning, 14(1), 16–39.
Moore, M. G. (1989). Editorial: Three types of interaction. The American Journal of Distance Education, 3(2), 1–6.
Richardson, J. C., & Ice, P. (2010). Investigating students' level of critical thinking across instructional strategies in online discussions. The Internet and Higher Education, 13(1-2), 52-59.
Sher, A. (2009). Assessing the relationship of student-instructor and student-student interaction to student learning and satisfaction in Web-based online learning environment. Journal of Interactive Online Learning, 8(2).
Smith, P. L., & Ragan, T.J. (2005). Instructional design, 3rd ed. Hoboken, NJ: Wiley.
Tawfik, A.A., Giabbanelli, P.J., Hogan, M., Msilu,F., Gill, A., & York, C.S. (2018). Effects of success v failure cases on learner-learner interaction. Computers & Education, 118, 120-132.