Courseware at Scale: Using Artificial Intelligence to Create Learning by Doing from Textbooks
Concurrent Session 7
Brief Abstract
Can you differentiate between AI-generated and human-authored questions? What if the answer is no? In this session, we will introduce the AI questions and the process that generates courseware from textbooks, learn from the faculty who used the courseware with students, and compare performance metrics of AI and human-authored questions.
Presenters
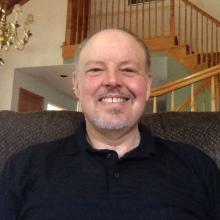
Extended Abstract
Session Topic
After the global pandemic disrupted 94% of students worldwide (UN, 2020), the online learning trend has only been accelerated, and digital learning resources are clearly here to stay. Yet with digital learning tools increasing exponentially, how do you determine what to use? New technology should not be used for the sake of its newness, but for its ability to apply research-based learning methods and scale those methods to learners everywhere. Even the most cutting-edge artificial intelligence technology must be harnessed for the purpose of helping students learn more effectively and efficiently online.
There are many different study techniques, but not all are equally effective (see Dunlosky et al., 2016). Courseware learning environments incorporate key learning science methods that are proven to help students learn effectively. For instance, organizing content into smaller lessons with integrated formative practice and targeted feedback are features that have been shown to increase learning gains while decreasing the time it took students to achieve them (Lovett et al., 2008). The learning by doing method of integrating formative practice with short sections of learning material has been shown to have six times the effect size on learning over just reading the text (Koedinger et al., 2015). This relationship between doing practice and learning is called the Doer Effect, and follow-up research has found it to be causal (Koedinger et al., 2016; 2018; Olsen and Johnson, 2019). Doing practice did not just correlate to better outcomes, but rather was shown to cause better outcomes. This method is simple, yet highly effective.
So what is stopping this learning by doing method from being incorporated into every digital learning resource? Time and cost. Writing questions is time intensive and requires content and assessment expertise, making the volume of questions needed for this type of learning by doing approach prohibitive for most content providers and faculty. The barriers are simply too high for most institutions to develop courseware at scale.
A promising emerging solution is to harness artificial intelligence (AI) to generate the formative practice questions needed for this method, and to transform e-textbooks into a courseware learning environment automatically. We utilize natural language processing and machine learning to read the textbook content, chunk it into smaller content lesson sections, align learning objectives to those lessons, and create formative practice questions for each lesson. These processes can be completed in less than an hour. By comparison, it typically takes hundreds of hours to do these same tasks manually, even with a skilled development team and a courseware authoring platform. This initial courseware transformation can then be augmented with additional assessments and adaptivity by the course designer or instructor if desired, maximizing the benefits to the student while minimizing the lift for developers and instructors. This AI process makes it possible to create courseware at scale, bringing a more effective digital learning environment to every student.
While the future possibilities are exciting, the practical application is happening now. This automatically generated courseware has been used as the learning resource in several university courses. Instructors are vital to the success of any learning resource for students (Van Campenhout & Kimball, 2021), so we will hear from expert, award-winning faculty in Psychology and Biology who have implemented this autogenerated courseware into their classrooms. Through a question and answer format, they will share their teaching and learning contexts, the creation and augmentation of the courseware, student responses, and their experience-based best practices for implementing this technology in the classroom. Faculty will also present anonymized course data on how students performed on exams and how they perceived the courseware experience.
New technology should always be thoroughly evaluated for its impact on student learning. When discussing AI-based solutions in particular, the question “How good is it?” is often asked. When we delve into what good means, it becomes clear the concern is if AI questions can be as good as human-authored questions. While good is a subjective construct, we can evaluate questions on a series of measurable performance metrics. The natural language processing and machine learning methods that created these formative questions were extensively tested and evaluated during their development, but the true test of their quality is in the results from natural student use. As both faculty here augmented the courseware further with more formative practice and adaptivity, we had a unique opportunity to compare questions used by the same students. Courseware with AI questions side-by-side with added human-authored questions, as was the case in these examples, provides a unique opportunity to compare these types of questions using performance metrics. Engagement measures the rate at which students chose to answer formative questions, difficulty is the first attempt accuracy on answered questions, and persistence is how often students continued to answer a question until they achieved the correct response. By using a mixed effects logistic regression model, these metrics were evaluated to determine if there were meaningful differences between the question types. Results of the analysis from these courses, in which the same students answered both AI-generated and human-authored questions, will be shared and discussed.
Interactivity
AI in education is an exciting topic, but also can raise many questions of its own. We will begin the session with a fun activity to engage the audience and show the output of the AI: lightning rounds of question classification. Using a mobile device clicker application, we will display questions and ask the audience to classify them. Each round will focus on a different classification metric (such as AI or human-authored, more or less difficult, etc.) and last approximately two minutes. The results will be revealed at the end of the activity. Participation prizes will also be awarded to audience members for engaging in the classification rounds.
The Takeaways
In this session, audience members will learn about the current state of automatic question generation and the AI methods that are currently transforming educational technology. Furthermore, attendees will learn how this technology was applied in the classroom from expert faculty members. The faculty panelists will share their learning contexts, teaching model, implementation practices, student reactions, and lessons learned. Finally, data analysis from real courses will be shared. A mixed effects logistic regression model compares AI and human-authored questions answered by the same students on three key performance metrics: engagement, difficulty, and persistence. While the use of AI in educational technology creates new avenues of possibilities, it is paramount that these new approaches be rigorously tested to ensure their benefit to student learning. Attendees will leave this session with an understanding of the role of AI in automatic question generation and automated courseware creation, the use of this technology in the classroom, and the results of rigorous analyses of the student data.
References
- Dunlosky, J., Rawson, K., Marsh, E., Nathan, M., & Willingham, D. (2013). Improving students’ learning with effective learning techniques: promising directions from cognitive and educational psychology. Psychol. Sci. Public Interest, 14(1), 4–58. https://doi.org/10.1177/1529100612453266
- Koedinger, K., Kim, J., Jia, J., McLaughlin, E., & Bier, N. (2015). Learning is not a spectator sport: doing is better than watching for learning from a MOOC. In: Learning at Scale, pp. 111–120. Vancouver, Canada. http://dx.doi.org/10.1145/2724660.2724681
- Koedinger, K., McLaughlin, E., Jia, J., & Bier, N. (2016). Is the doer effect a causal relationship? How can we tell and why it’s important. Learning Analytics and Knowledge. Edinburgh, United Kingdom. http://dx.doi.org/10.1145/2883851.2883957
- Koedinger, K. R., Scheines, R., & Schaldenbrand, P. (2018). Is the doer effect robust across multiple data sets? Proceedings of the 11th International Conference on Educational Data Mining, EDM 2018, 369–375.
- Kurdi, G., Leo, J., Parsia, B., Sattler, U., & Al-Emari, S. (2020). A Systematic Review of Automatic Question Generation for Educational Purposes. International Journal of Artificial Intelligence in Education, 30(1), 121–204. https://doi.org/10.1007/s40593-019-00186-y
- Lovett, M., Meyer, O., & Thille, C. (2008). The Open Learning Initiative: Measuring the Effectiveness of the OLI Statistics Course in Accelerating Student Learning. Journal of Interactive Media in Education, p.Art. 13. DOI: http://doi.org/10.5334/2008-14
- Olsen, J., & Johnson, B. G. (2019). Deeper collaborations: a finding that may have gone unnoticed. Presented at the IMS Global Learning Impact Leadership Institute, San Diego, CA
- UN. (2020). Policy Brief: Education during COVID-19 and beyond. United Nations. https://www.un.org/development/desa/dspd/wp-content/uploads/sites/22/2020/08/sg_policy_brief_covid-19_and_education_august_2020.pdf
- Van Campenhout, R. & Kimball, M. (2021). At the intersection of technology and teaching: The critical role of educators in implementing technology solutions. IICE 2021: The 6th IAFOR International Conference on Education.